Generative AI is changing industries with time. It excels brilliantly in various contexts and multiple tasks, with higher accuracy and speed than humans. However, because of generative AI models’ unpredictable and occasional errors, which vary from eccentric to offensive, few users and businesses are reluctant to embrace this all-rounder technology completely. This caution is completely justified. In high-exposure or high-stakes tasks, even small errors could cause serious consequences.
Numerous top-rated practices are available to GenAI adopters that enhance domain expertise, reliability, and accuracy. Additionally, they boost trust in AI systems, allowing organizations to maximize their profits from generative AI—the most promising and gradually trending AI system in retrieval-augmented generation (RAG). With appropriate model training, integration, and source materials, RAG is composed to mitigate, if not correct, a few of generative AI’s most stubborn issues.
Understanding RAG
Retrieval-augmented generation is a two-step procedure involving generation and retrieval. In the retrieval phase, users suggest a query, which initiates a relevancy search in the external documents. This system then collects snippets of data relevant to the user query and includes them in the prompt within the context window. The additional data and the prompt are fed from the GenAI model, which processes a response based on pre-built knowledge and the RAG search information.
The RAG system executes a nearest-neighbor search to find database items closest to the user’s query. The retrieved data is then attached to the prompts through the context window and utilized to create a quality response.
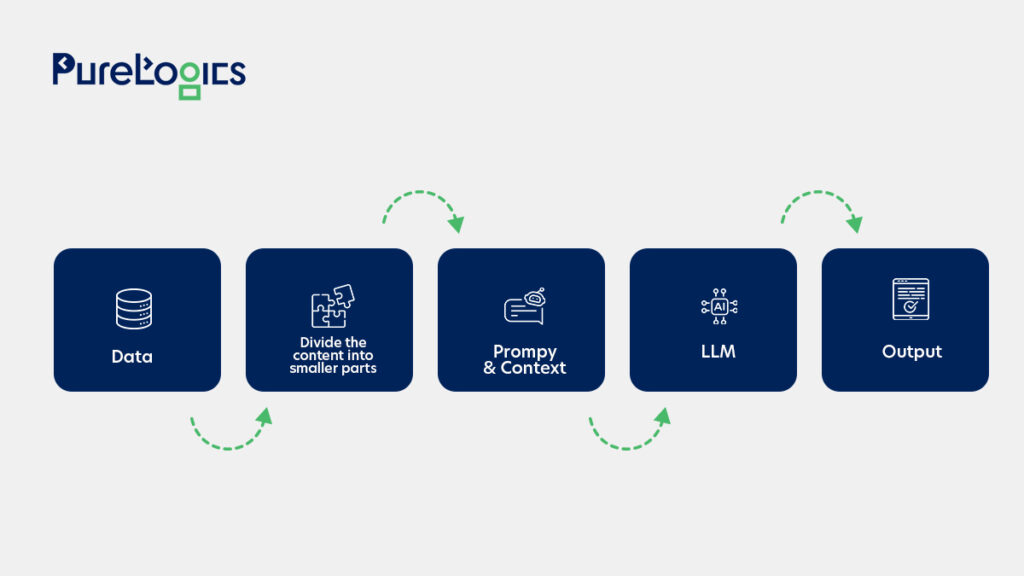
Core Elements of RAG Framework
Knowledge Graphs
Such graphs present enriched data, revealing connections and relations that base the AI’s responses on accurate information.
Contextual Data Enrichment
Using business-oriented ontologies and taxonomies, RAG assists the AI in understanding the meaning and context behind the data.
Prompt Enhancement
After receiving a user query, the knowledge graph builds the prompt, offering context to generate factual answers.
Response Validation
All AI responses are cross-checked against the knowledge model to ensure reliability and accuracy before being offered to the user.
Challenges Addressed by RAG
The perks of retrieval-augmented generation are wide-ranging and powerful:
Minimized Hallucinations
RAG lets static, enormous LLMs with obsolete training data access specialized or new information to counter user queries. This massively minimizes hallucinations by exposing holes in the base model’s knowledge and providing it with the context to produce accurate responses.
Latest Information
RAG controls the time break from training data by giving the model access to real-time or current information about topics and events after the end of model training. This also eliminates hallucinations and boosts the relevance and accuracy of responses.
Seamless Updates
The RAG framework detours the need for time-intensive and costly retraining and updating of base models. Databases can be updated by including fresh documents such as policies, new products, and procedures or by organically accessing the Internet.
Source Citations
It gives much-required visibility into the origins of generative AI responses. Any response that points to external data offers source citations, empowering for fact-checking and direct verification.
Domain-specific Knowledge
RAG is an efficient and effective way to augment foundation models with domain-related data. Vector databases are created relatively cheaply and at scale since they don’t need labeled SMEs or datasets. This makes RAG the best available approach for model specialization to date, compared with proprietary model building, prompt engineering, and refining.
Struggling to Keep Up with Generative AI Advancements?
Discover how our generative AI solutions can transform your business for the future.
RAG Frameworks: Training and Integration Strategies
The model training should be as precise and comprehensive as possible for a retrieval-augmented generation framework to offer accurate, comprehensive responses. While AI can automate and facilitate some parts of the training procedure, the base training tasks for these frameworks need qualified domain-expert annotators.
To upskill and integrate a RAG framework, human domain experts should assist the model through numerous stages of responses and search queries by offering high-quality examples. These include parsing user prompts, processing search queries to external sources, identifying retrieved data for completeness and relevance, and composing accurate, ethical, and well-written responses. The model’s performance depends on how qualified the model trainers are, what data sources and training methods they are using, etc.
RAG and Generative AI- The Future
Technology, no matter as pervasive and disruptive as generative AI, will have growing pains, but regardless, GenAI has the potential to do great work in the future.
The current or possible challenge for organizations is finding ethical and safe generative AI integration practices and adoption. This involves staying current with technological changes that improve the trustworthiness and reliability of AI outputs. RAG can address multiple ongoing limitations of generative AI by increasing transparency and accuracy and reducing hallucinations. When advanced ethical safeguards and technical processes match LLMs’ computing tendency, generative AI will become a formidable engine of better change.
About PureLogics
PureLogics has 18+ years in offering quality generative AI solutions. Reinvent your enterprise efficiency and workflows with our robust generative AI capabilities. We have expert GenAI engineers ready to give you top-tier generative AI consultancy in various industries. Give us a call today!