Automated Machine Learning (AutoML) is reshaping the world of artificial intelligence (AI), bringing us closer to a reality where “AI creates AI.” As this concept gains momentum, the importance of AutoML platforms is becoming undeniable.
AutoML is turning AI into more accessible technology by letting companies discover advanced machine learning approaches without requiring in-depth data science expertise. With the market for AutoML projected to grow significantly, reaching $7.35 billion by 2028 and expanding at a 44.9% annual growth rate, its impact is clear.
One of the biggest hurdles for AI adoption has been the need for more skilled professionals. AutoML helps close this gap by automating complicated tasks like feature engineering, model selection, and hyperparameter tuning. This speeds up AI model development and slashes the costs and time needed for machine learning projects.
Industries are already seeing the benefits of AutoML. Companies using these platforms have reported faster model development and quicker deployments. AutoML is not just automating tasks—it’s revolutionizing how organizations tackle problems and make decisions.
The Value of AutoML in Modern AI Development
Here are a few compelling reasons to emphasize the importance of AutoML in modern AI development:
Standardization of Machine Learning
AutoML allows machine learning to a wider audience, not just experienced data scientists. This democratization allows organizations without deep ML expertise to harness the power of AI and make data-driven decisions.
Time Saving and Efficiency
Traditional machine learning can be time-intensive, requiring manual steps like feature engineering, model selection, and hyperparameter tuning. AutoML automates these processes, reducing the time needed to build and deploy models.
Solving the Skills Gap
With a global shortage of skilled data scientists and ML experts, AutoML platforms help fill this gap. They allow professionals with basic knowledge of machine learning to develop sophisticated models without needing deep expertise.
Cost Reduction
By automating many parts of the machine learning pipeline, AutoML can reduce the need for large teams of specialized data scientists, which helps lower organizational costs.
Scalability
AutoML solutions are built to tackle massive datasets and can seamlessly scale machine learning apps across a company, automatically adapting to different types of issues.
Enhanced Model Performance
AutoML platforms can explore a wide range of models and hyperparameters systematically, often delivering better-performing models than those developed manually, especially for less-experienced practitioners.
Rapid Prototyping
AutoML facilitates the quick development of prototype models, allowing faster iteration and experimentation in machine learning projects.
Interpretability and Transparency
Few AutoML platforms provide tools to analyze model decisions, addressing problems related to the “black box” nature of complicated ML models.
Retraining and Maintenance
AutoML platforms often have streamlined processes for maintaining and retraining models, ensuring they remain accurate and useful over time.
Key Components of AutoML
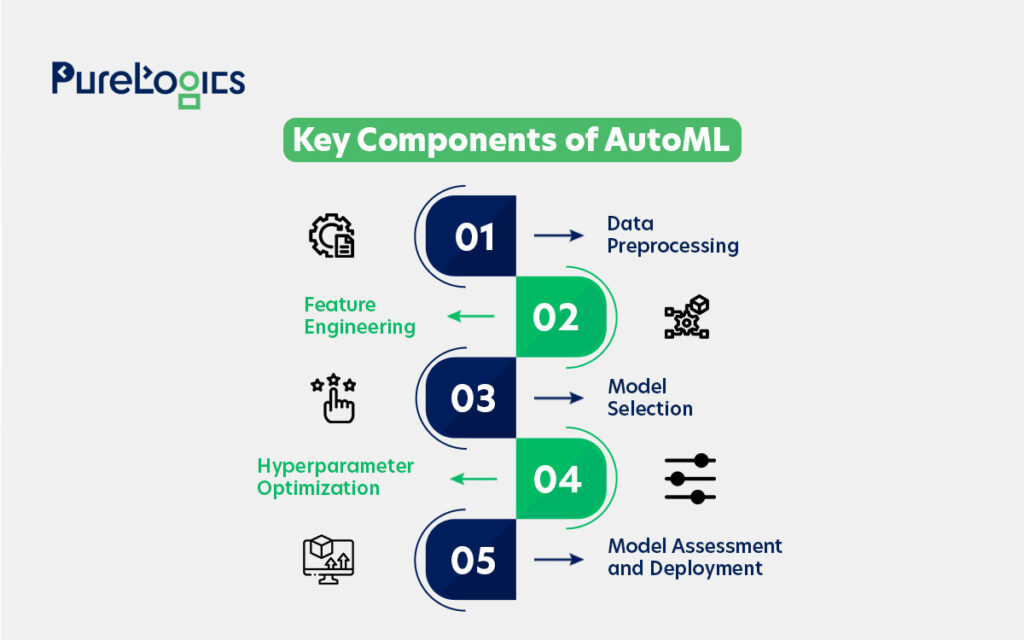
Automated Machine Learning (AutoML) simplifies the application of machine learning by automating several essential tasks that typically require a high level of expertise. These factors include feature engineering, data preprocessing, hyperparameter optimization, model selection, and model deployment and evaluation. Every component is key in developing accurate, efficient, and effective ML models. Here’s a breakdown:
Data Preprocessing
Data preprocessing is the cornerstone of any machine learning project. The performance of a model depends heavily on the quality and structure of the data fed into it. AutoML automates this critical step, ensuring the data is cleaned, transformed, and ready for analysis without requiring much human input.
Feature Engineering
Feature engineering involves selecting, modifying, or creating new features from raw data to enhance model performance. This task is often both creative and time-consuming. AutoML automates the process by pinpointing the most relevant features and generating new ones to boost the model’s predictive power.
Model Selection
The right machine learning algorithm is crucial for obtaining accurate and efficient results. AutoML automates the process by comparing multiple algorithms and selecting the one that best suits the data, taking much of the guesswork out of the equation.
Hyperparameter Optimization
Hyperparameter optimization is fine-tuning a model’s settings to maximize its performance. Each machine learning algorithm has specific hyperparameters that dictate how it learns from the data. AutoML automates this process, adjusting these settings to significantly improve model accuracy and efficiency.
Model Assessment and Deployment
After a model is built, it must be evaluated to ensure it works well with unseen data. Once it passes the evaluation, the model can be deployed for real-world use. AutoML simplifies both of these tasks, making it easier to assess the model’s performance and quickly implement it in real-world applications.
Together, these components of AutoML streamline the entire machine-learning pipeline, enabling faster, more accurate, and efficient model development with minimal human effort.
Unlock the potential of AutoML today.
Simplify AI development and drive your business forward with PureLogics.
The Concept of “AI Creating AI”
AutoML platforms are revolutionizing AI by automating complex tasks, allowing AI to design, fine-tune, and deploy other AI models. This idea of “AI creating AI” reduces the requirement for human involvement in creating models, making advanced machine-learning techniques more accessible to everyone.
At its core, AutoML is a form of AI using machine learning algorithms to decide how best to create and improve other models. This meta-level AI continuously learns and adapts, improving its effectiveness with each project. As a result, it speeds up model creation and can uncover innovative approaches and model designs that even experienced data scientists might overlook.
As these systems advance, they can expand the boundaries of what’s possible in machine learning and AI, leading to breakthroughs in both speed and innovation.
How PureLogics Handle Enterprise Challenges with AutoML
PureLogics uses AutoML to tackle complex business challenges efficiently. By leveraging leading platforms such as AWS, Azure, and Google AutoML, PureLogics simplifies AI development, making it accessible to businesses across various sectors. Here’s a closer look at how PureLogics uses AutoML:
Advanced-Data Visualization
PureLogics uses sophisticated visualization techniques to turn complex data into clear, actionable insights. They create interactive dashboards and reports customized to each client, helping stakeholders identify trends, patterns, and outliers for smarter decision-making.
Intelligent Feature Engineering & Data Preprocessing
PureLogics automates the data preparation, handling missing values, selecting important features, and transforming data into formats that machine learning algorithms can easily use. Their in-house tools ensure the data is perfectly prepared for model training, leading to more accurate AI solutions.
Automated Model Selection & Development
PureLogics employs AutoML services like AWS SageMaker Autopilot, Azure AutoML, and Google Cloud AutoML to quickly develop and compare thousands of models. This approach ensures that the best model for a specific problem is chosen in a fraction of the time traditional methods would require.
Detailed Model Documentation
PureLogics provides detailed, easy-to-understand documentation for every model. Their reports cover model architecture, data preprocessing steps, performance metrics, limitations, and usage instructions. This transparency helps teams collaborate effectively and meet regulatory requirements.
Efficient & Scalable Deployment
Using cloud platforms like AWS, Azure, and Google Cloud, PureLogics ensures that AI models are deployed smoothly and can scale to meet business demands. This allows businesses to integrate AI into their systems with minimal disruption.
Ongoing Model Improvements & Monitoring
PureLogics sets up continuous monitoring for deployed models, quickly detecting any drops in performance. This allows them to make timely adjustments, ensuring models stay effective over time.
Industry-focused Solutions
By tailoring their approach to different industries, PureLogics ensures that their AI solutions align with industry standards and regulations, providing customized solutions that meet specific business needs.
Through these AutoML-driven strategies, PureLogics accelerates the development of AI, reduces time-to-market, and helps businesses unlock the full potential of AI. Their commitment to thorough model documentation and advanced data visualization ensures that AI solutions are not only accurate, transparent, and easy to interpret.
Concluding Thoughts
AutoML is a game-changing step forward for AI, paving the way for a future where AI is accessible to everyone. By removing the complexity and expertise barriers, AutoML is democratizing AI.
Use the power of AutoML with PureLogics. Let us turn your data into actionable insights and speed up your AI journey. Partner with us to innovate and drive your business forward!