Information has turned into an essential commodity in numerous sectors, particularly with the use of the World Wide Web and mobile devices. This data assists businesses to get to know their users, create plausible frameworks for progression, or deliver their services more effectively.
Data is valuable in healthcare as they are primarily chosen and selected in their collection. The benefits of the above data can be seen when used by the health systems, providers, and patients in enhancing the health delivery systems and outcomes if only enhanced with high analytical methods such as AI.
Today there is much focus on the development of healthcare AI systems, and below are key reasons why. Now, it is high time that we explored some of the major applications of AI and its ancillary uses in healthcare.
Use of AI in Healthcare
Clinical Decision Support
Clinical decision support systems are indispensable work aids of the health care sector to improve the quality of care and reduce risks. These systems are now being used in clinical decision making given the development in the technologies like artificial intelligence (AI) and machine learning (ML).
In the early days of Assessment, CDS tools were analogously often single tools far removed from the processes in the clinics. Modern CDS implementation involves integrating it as a component of an EHR to enhance the systems’ placement and operations at the point of patient care.
AI improves this integration since it can also consider data from the EHR and from other sources. AI-based systems surpass the traditional techniques in terms of speed and increased productivity through large volumes of data that in turn offers enhanced results to support clinical practice.
CDS tools can embed the technology of risk stratification and predictive analysis since AI has better pattern recognition. This enables development of more specific recommendations on the treatment, which are important regarding chronic disorders.
Clinical Trials & Medical Research
Health research therefore plays an important role in shaping the healthcare sector by developing new processes, procedures, treatments and therapies. But, this research, particularly clinical trials, requires adequate capital, time, and other resources for the process.
With the help of AI tools, data can be collected and organized faster and more effectively, and trial enrollment can also be improved, which is why AI is very useful in medical research.
These technologies assist in achieving faster clinical trials as they contribute in designing efficient trials, expediting eligibility checks, and implementing effective recruitment processes. Moreover, AI is used to help sort through large amounts of clinical trial data, identify trends, and predict outcomes, and come up with treatment plans based on patient data.
In addition to research, various AI technologies are seen to have benefits in the patient experience.
Drug Discovery & Development
Pharmaceutical research for the discovery, development and making of drugs have contributed to the creation of new medical therapies for many health complications. This integration of AI to these programs and other technologies is still transforming the pharmaceutical industry.
It is positive to note that with the help of AI as well as other technologies, important obstacles in drug discovery and development can indeed be overcome. The gap between the potential of AI and the current industry adoption can be attributed to the increased usage of AI and ML in enhancing drug manufacturing through better process control, predictive maintenance, and quality assurance. Such abilities can determine data patterns that might go unnoticed by human analysts, making them useful.
This was in a research which occurred in June 2023 titled, “AI-enabled prospective drug design” published in Science Advances. Specifically, the study showed that a generative AI model could create new molecules that could potentially inhibit SARS-CoV-2, the virus behind COVID-19. The use of the AI tool was easy and the model simulated the ability to identify drug-like molecules that interact with two Covid-19 proteins involved in the transmission of the virus.
AI models are vital not only for drug discovery but also for turning EHRs into knowledge that can be relied on. This capability majors in the prospect of boosting both fields to another new level.
Hospital Management
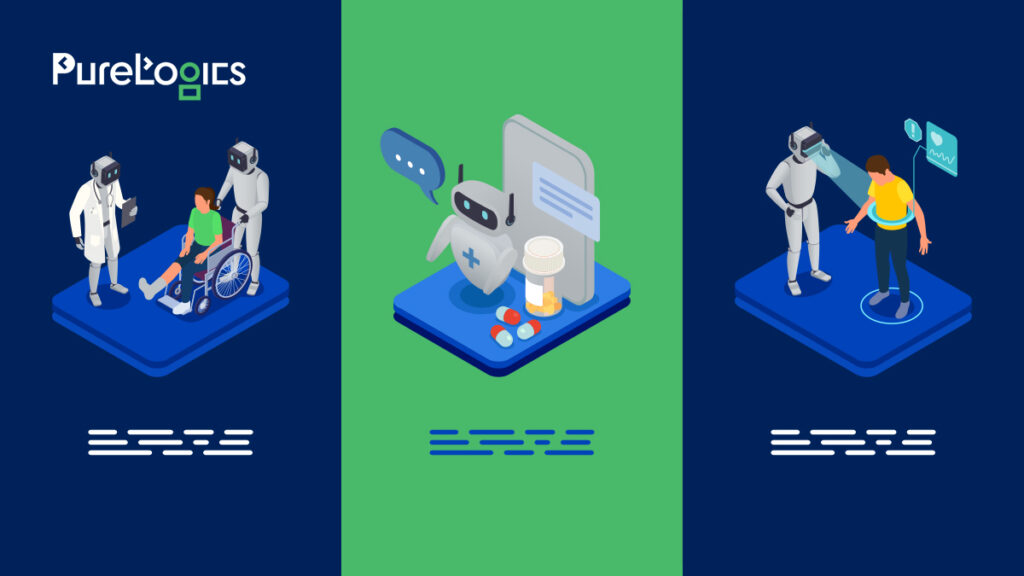
For the administration of health systems and the distribution of revenues, key components of artificial intelligence in the region are necessary. Evaluating the efficiency and congestion has a greater influence on the financial performance and delivery of quality outcomes in a health care setting.
However, being in charge of all the necessary resources is not an easy job and that is why the varieties of health systems address their data analytics needs to AI. Inventory management remains a thorny issue depending on the capacity management of the hospitals given that they have not enough staff and the Covid pandemic has worsened the ways hospitals manage the aforementioned issues like; surgery timetable.
These issues can only be resolved through the coordination of health systems with considerations of available staffing and surgeon choices and preferences. The strategies presented above indicate that there is a blend of factors to consider here, and data analytics and AI can assist in working through this. These differences are also achieved from the utilization and the scheduling of the ORs, leading to instances whereby OR equipment lies idle, there are empty ORs, or the surgeon is unable to secure OR block time.
Other branches of AI in healthcare have also benefited from the growth of AI and ML technologies, which have improved fields like medical imaging by increasing the productivity of both the care recipient and provider.
Medical Imaging
Diagnostics and pathology depend heavily on medical imaging, but making sense of these images can be highly challenging even for a professional. To overcome this challenge, advanced imaging analytics, mostly driven by Artificial Intelligence, has come into the scene.
A work done by Johns Hopkins researchers in March 2024, later published in Communications Medicine, showed that when clinicians in emergency rooms are trained to use a deep neural network-based automated detection tool that uses lung Ultrasound Images to diagnose COVID-19 it could be of help.
The advantage of this tool is the visualization of B-lines, acute, vertical image features of pulmonary lesions indicating COVID-19 infection. The application of this model should therefore indicate that a similar strategy could be used in other severe disorders, such as heart failure, to facilitate early diagnosis at healthcare’s early point of care.
The researchers noted that such capability would be very useful to support ED clinicians when they are under many patients, for instance at the start of the COVID 19 pandemic. It could also be SC installed in wearable technologies and other wireless technologies for better management of remote patients.
Patient Engagement
Interpersonal communication is one of the major components of patient satisfaction and involvement. EHRs enable this through secure messaging to enable information exchange between patients and providers when it is convenient for both parties. However, EHR can be inundated with messages, which may exacerbate clinician burnout because some questions require more time to answer.
AI chatbots present an opportunity to solve this problem as patients are received accordingly and taken through the right channel to the right resource. For example, a health system may employ a chatbot in managing patient calls with such simple issues addressed such as directions to a hospital parking lot.
In addition to this, these artificial intelligence tools also serve clinical needs where using patient symptoms as key input to recommend care.
AI patient engagement tools can also extend to patient communication tools that rely on clinical risk assessment information or that work to translate content for a patient-facing patient portal. Sometimes, these tools provide recommendations on the engagement processes that need to be followed or offer other significant results.
Predictive Analysis
Over the recent past, analytics has enabled artificial intelligence to perform patient care more proactively in health facilities. Outcome prediction is valuable in the creation of essential therapies and directing patient care in the current environment of value-based practice.
Predictive analytics, in turn, requires that numerous are processed and analyzed by stakeholders, from a variety of sources, including accurate data. Most of the predictive modeling tools use AI to manage this data; these tools are not only advantageous but also have huge application requirements.
It improves clinical decision support, population health management, and value-based care delivery; Artificial intelligence in the area of predictive analytics is progressing further in the field of healthcare. These efforts are facilitated by the use of AI-based risk stratification that helps in altering harm-causing results in patients who are at high-risk for such occurrences.
Along with machine learning, the methods of remote patient monitoring have been studied actively with the help of AI tools.
Future of AI in Healthcare
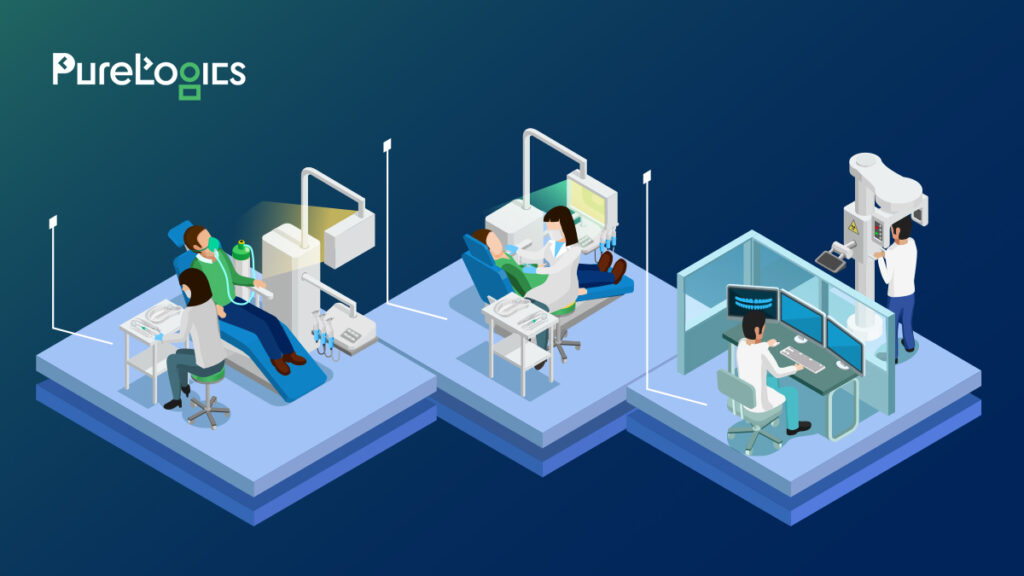
With time, AI is expected to become an even more core part of the healthcare industry. Thus, AI, specifically machine learning, which is currently at the core of personalized medicine, is more of a leap forward in a healthcare system. It is noted that initial efforts to propose diagnosis as well as treatment or management strategies are still a problem in the current AI systems, but certainly, the field stands to improve in future.
Given the progress being made in deep learning and other forms of AI in analyzing images, there may very soon be a time where machines will be diagnosing most of the images that are spotted in radiology and pathology departments.
The most significant issue related to artificial intelligence implementation in healthcare management is not the capacities on the technological levels themselves, but their applicable application into clinical practices. To become more widely adopted, AI systems must also be cleared by the regulation and government to be used in patient care, require understanding of how Electronic Health Record systems work, to have the same product behave similarly, taught to clinicians, and to be funded by public and private insurance payers along with being updated regularly.
The use of such smart technologies as chatbots is on the rise and contributes to patients and their progress by notifying patients about their health and guiding through various conversations in natural language.
About PureLogics
To develop AI technologies for the healthcare sectors, probably the most imperative factor is the enrolment of technology-wise and industry-wise skilled teams. PureLogics is a leading technology solutions provider specialized in the IT field with 20 years of experience. It gives PureLogics a competitive edge and makes it capable of offering the insights and solutions necessary for success in the healthcare industry with a team of the best professionals across the globe. Give us a call today!